Demystifying AI and Analytics
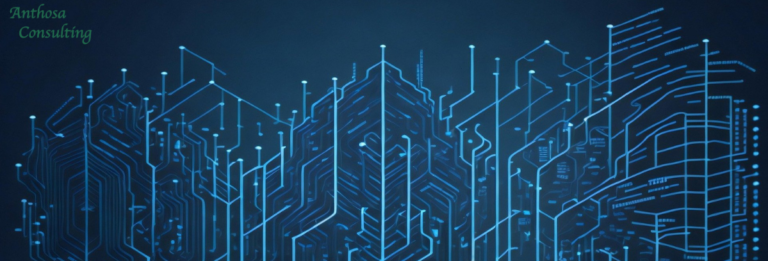
Empowering the Future with Analytics to Generative AI and Dispelling Unfounded Fears
In the ever-evolving landscape of technology, Artificial Intelligence (AI) and analytics have emerged as transformative forces. From descriptive insights to predictive forecasting and prescriptive decision-making, the realm of analytics has expanded. When coupled with AI, these capabilities are further augmented, unlocking immense potential across various domains. This article aims to demystify the concepts of descriptive, predictive, and prescriptive analytics while exploring their relationship with AI and machine learning. We will also delve into the fascinating world of Generative AI and address the unfounded fears surrounding these cutting-edge technologies, ultimately revealing how they empower the future with knowledge.
Descriptive, Predictive, and Prescriptive Analytics: The Foundation
Descriptive analytics forms the bedrock of data analysis, providing a retrospective view of past events and trends. By summarising historical data, businesses gain valuable insights into their performance, customer behaviour, and market trends. However, to stay competitive, organisations must look beyond the past and adopt predictive analytics.
Predictive analytics leverages statistical models and machine learning algorithms to anticipate future trends and events. By identifying patterns in historical data, it enables businesses to make informed decisions and capitalise on opportunities. Machine learning, a subset of AI, plays a pivotal role in predictive analytics. Supervised learning, where the algorithm is trained on labelled data, and unsupervised learning, which finds patterns in unlabelled data, are prominent techniques.
Prescriptive analytics takes things a step further by recommending optimal actions based on predictive insights. It combines historical data, predictive models, and business rules to provide actionable recommendations. Prescriptive analytics is closely linked to reinforcement learning, an AI paradigm where an agent learns to make decisions by interacting with its environment and receiving feedback in the form of rewards. This type of learning enables AI systems to adapt and improve their decision-making over time.
Artificial Intelligence and Machine Learning: The Synergy
Artificial Intelligence, a broader concept, encompasses machine learning and other techniques that simulate human intelligence. Machine learning, on the other hand, is a subset of AI focused on training algorithms to improve their performance on a specific task. Supervised learning is widely used for classification and regression tasks, where the algorithm learns from labelled data to make predictions on unseen instances. This approach is useful for applications like image recognition, sentiment analysis, and speech recognition.
Unsupervised learning, on the contrary, is employed for clustering and dimensionality reduction. The algorithm seeks to find patterns or groupings in the data without any predefined labels. This technique proves valuable in customer segmentation, anomaly detection, and recommendation systems.
Reinforcement learning, as previously mentioned, enables AI systems to learn through trial and error, ultimately making optimal decisions in dynamic environments. This approach is particularly promising in applications like autonomous vehicles, robotics, and game-playing.
Generative AI: Fostering Creativity and Imagination
Generative AI is a revolutionary branch of AI that takes creativity to unprecedented levels. Unlike traditional AI, which focuses on problem-solving and decision-making, generative AI is all about producing new content. This technology relies on generative models like Generative Adversarial Networks (GANs) and Variational Autoencoders (VAEs) to create content such as images, music, text, and even entire videos.
GANs consist of two neural networks – a generator and a discriminator – that play a game of cat and mouse. The generator creates synthetic data and aims to deceive the discriminator into believing it’s real. Through iterative training, GANs become proficient at generating highly realistic and original content.
VAEs, on the other hand, focus on learning latent representations of data and can be used for creative tasks such as image-to-image translation, style transfer, and even generating new artworks.
Generative AI is a double-edged sword, as its potential for creativity is unparalleled, but it also raises concerns about the authenticity of digital content and potential misuse for creating fake information..
Unfounded Fears of AI: Separating Fact from Fiction
As AI advances, so do concerns and misconceptions about its impact. One prevalent fear is that AI will replace human jobs on a massive scale. While automation may affect certain job roles, AI also creates new opportunities, necessitating a shift in the workforce and fostering the development of new skills.
Another misconception is the idea of AI becoming uncontrollable and dominating humanity, reminiscent of science fiction scenarios. In reality, AI systems are designed and programmed by humans, and ethical considerations remain paramount in their development.
Furthermore, fears of AI developing emotions or consciousness are unfounded. AI lacks the biological structure and subjective experience necessary for emotions or consciousness to emerge.
Conclusion
The concepts of descriptive, predictive, and prescriptive analytics, when combined with AI and machine learning, have the potential to revolutionise decision-making and empower organisations across the globe. Generative AI opens up new frontiers of creativity and imagination while debunking unfounded fears surrounding AI is essential for embracing these transformative technologies responsibly. As we continue to explore the possibilities of AI and analytics, it is crucial to approach these advancements with confidence, fostering a future enriched by knowledge and innovation.